Prof. Dr.-Ing. Erhardt Barth
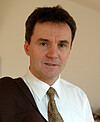
Stellvertretender Direktor
Institut für Neuro- und Bioinformatik
Ratzeburger Allee 160 (Geb. 64)
23562 Lübeck
Email: | erhardt.barth(at)uni-luebeck.de |
Phone: | +49 451 3101 5503 |
Fax: | +49 451 3101 5504 |
Current research interests
Main research interests are in Computer Vision and Machine Learning. A further interest is in biological vision, which is used as a source of inspiration for solving computer vision problems and for building enhanced vision systems that can compensate the strengths and weaknesses of both human and computer vision.
Brief biography
Prof. Barth leads the research on human and machine vision at the INB. He obtained his Ph.D in Electrical Engineering from the Technical University of Munich in 1994, was a Research Associate at the Department of Communications Engineering in Munich and a Visiting Fellow at the Department of Computer Science, Melbourne University, Australia, where he was supported by the Gottlieb-Daimler and Karl-Benz Foundation. He then was a researcher at the Department of Medical Psychology, University of Munich, and a Klaus-Piltz fellow at the Institute for Advanced Study in Berlin. In 1997/98 he was a member of the NASA Vision Science and Technology Group at NASA Ames, Moffet Field, California. In May 2000 he received a Schloessmann Award from the Max-Planck Gesellschaft. Since then, he initiated and conducted a number of basic and applied research projects, and started a few companies (e.g. ARTTS, GazeCom, PRC, gestigon).
Google Scholar
Publications
2022
Automated assessment of immunofixations with deep neural networks, Journal of Laboratory Medicine , vol. 46, no. 5, pp. 331--336, 2022. De Gruyter.
Datei: | |
Bibtex: | ![]() @article{ThKlMaGrKaBaKrMa22, title={Automated assessment of immunofixations with deep neural networks}, author={Thiemann, Christian and Klitzke, Britta and Martinetz, Philipp and Gr{\"u}ning, Philipp and K{\"a}ster, Thomas and Barth, Erhardt and Kramer, Jan and Martinetz, Thomas}, journal={Journal of Laboratory Medicine}, volume={46}, number={5}, pages={331--336}, year={2022}, publisher={De Gruyter}, url={https://www.degruyter.com/document/doi/10.1515/labmed-2022-0078/pdf} } |
FP-nets as novel deep networks inspired by vision, Journal of Vision , vol. 22, no. 1, pp. 8--8, 2022. The Association for Research in Vision and Ophthalmology.
Datei: | |
Bibtex: | ![]() @article{GrMaBa22, title={FP-nets as novel deep networks inspired by vision}, author={Gr{\"u}ning, Philipp and Martinetz, Thomas and Barth, Erhardt}, journal={Journal of Vision}, volume={22}, number={1}, pages={8--8}, year={2022}, publisher={The Association for Research in Vision and Ophthalmology}, url={https://www.inb.uni-luebeck.de/fileadmin/files/publications/inb-publications/pdfs/GrMaBa22.pdf} } |
TEVR: Improving Speech Recognition by Token Entropy Variance Reduction, 2022. arXiv.
DOI: | 10.48550/ARXIV.2206.12693 |
Datei: | 2206.12693 |
Bibtex: | ![]() @misc{KrBa22, author = {Hajo Nils Krabbenh{\"o}ft and Erhardt Barth}, title = {{TEVR}: {I}mproving {S}peech {R}ecognition by {T}oken {E}ntropy {V}ariance {R}eduction}, publisher = {arXiv}, year = {2022}, keywords = {Computation and Language (cs.CL), Sound (cs.SD), Audio and Speech Processing (eess.AS), FOS: Computer and information sciences, FOS: Computer and information sciences, FOS: Electrical engineering, electronic engineering, information engineering, FOS: Electrical engineering, electronic engineering, information engineering, F.2.1; I.2.6; I.2.7}, doi = {10.48550/ARXIV.2206.12693}, url = {https://arxiv.org/abs/2206.12693}, copyright = {Creative Commons Attribution 4.0 International} } |
2021
An AI-based Framework for Diagnostic Quality Assessment of Ankle Radiographs, in Proceedings of the Fourth Conference on Medical Imaging with Deep Learning , Heinrich, Mattias and Dou, Qi and de Bruijne, Marleen and Lellmann, Jan and Schläfer, Alexander and Ernst, Floris, Eds. PMLR, 07-.2021. pp. 484--496.
Datei: | |
Bibtex: | ![]() @inproceedings{MaLaSiSiBiKaBaBaMa21, title = {An {AI}-based Framework for Diagnostic Quality Assessment of Ankle Radiographs}, author = {Mairh{\"o}fer, Dominik and Laufer, Manuel and Simon, Paul Martin and Sieren, Malte and Bischof, Arpad and K{\"a}ster, Thomas and Barth, Erhardt and Barkhausen, J{\"o}rg and Martinetz, Thomas}, booktitle = {Proceedings of the Fourth Conference on Medical Imaging with Deep Learning}, pages = {484--496}, year = {2021}, editor = {Heinrich, Mattias and Dou, Qi and de Bruijne, Marleen and Lellmann, Jan and Schläfer, Alexander and Ernst, Floris}, volume = {143}, series = {Proceedings of Machine Learning Research}, month = {07--09 Jul}, publisher = {PMLR}, pdf = {https://proceedings.mlr.press/v143/mairhofer21a/mairhofer21a.pdf}, url = {https://www.inb.uni-luebeck.de/fileadmin/files/publications/inb-publications/pdfs/MaLaSiSiBiKaBaBaMa21.pdf}, abstract = {The quality of radiographs is of major importance for diagnosis and treatment planning. While most research regarding automated radiograph quality assessment uses technical features such as noise or contrast, we propose to use anatomical structures as more appropriate features. We show that based on such anatomical features, a modular deep-learning framework can serve as a quality control mechanism for the diagnostic quality of ankle radiographs. For evaluation, a dataset consisting of 950 ankle radiographs was collected and their quality was labeled by radiologists. We obtain an average accuracy of 94.1%, which is better than the expert radiologists are on average.} } |
Bio-inspired Min-Nets Improve the Performance and Robustness of Deep Networks, in SVRHM 2021 Workshop NeurIPS , 2021.
Datei: | |
Bibtex: | ![]() @inproceedings{GrBa21, title={Bio-inspired Min-Nets Improve the Performance and Robustness of Deep Networks}, author={Gr{\"u}ning, Philipp and Barth, Erhardt}, booktitle={SVRHM 2021 Workshop NeurIPS}, year={2021}, url={https://www.inb.uni-luebeck.de/fileadmin/files/publications/inb-publications/pdfs/GrBa21.pdf} } |
COVID-Nets: deep CNN architectures for detecting COVID-19 using chest CT scans, PeerJ Computer Science , vol. 7, pp. e655, 2021.
DOI: | 10.7717/peerj-cs.655 |
Datei: | peerj-cs.655 |
Bibtex: | ![]() @article{AlLiAbBaMa21, author={Hammam Alshazly and Christoph Linse and Mohamed Abdalla and Erhardt Barth and Thomas Martinetz}, title = {{COVID-Nets}: deep {CNN} architectures for detecting {COVID-19} using chest {CT} scans}, journal = {PeerJ Computer Science}, volume={7}, pages={e655}, year={2021}, doi = {10.7717/peerj-cs.655}, url = {https://doi.org/10.7717/peerj-cs.655} } |
Direct Inference of Cell Positions using Lens-Free Microscopy and Deep Learning, in Medical Imaging with Deep Learning , 2021. pp. 219--227.
Datei: | |
Bibtex: | ![]() @inproceedings{GrNeHeSoBa21, title={Direct Inference of Cell Positions using Lens-Free Microscopy and Deep Learning}, author={Gr{\"u}ning, Philipp and Nette, Falk and Heldt, Noah and de Souza, Ana Cristina Guerra and Barth, Erhardt}, booktitle={Medical Imaging with Deep Learning}, pages={219--227}, year={2021}, organization={PMLR}, url={https://www.inb.uni-luebeck.de/fileadmin/files/publications/inb-publications/pdfs/GrNeHeSoBa21.pdf} } |
Explainable COVID-19 Detection Using Chest CT Scans and Deep Learning, Sensors , vol. 21, no. 2, pp. 455, 2021. Multidisciplinary Digital Publishing Institute.
Datei: | |
Bibtex: | ![]() @article{AlLiBaMa21, author={Hammam Alshazly and Christoph Linse and Erhardt Barth and Thomas Martinetz}, title = {Explainable {COVID-19} {D}etection {U}sing {C}hest {CT} {S}cans and {D}eep {L}earning}, journal = {Sensors}, volume={21}, number={2}, pages={455}, year={2021}, publisher={Multidisciplinary Digital Publishing Institute}, url = {https://www.mdpi.com/1424-8220/21/2/455}, url = {https://www.inb.uni-luebeck.de/fileadmin/files/publications/inb-publications/pdfs/AlLiBaMa21.pdf} } |
FP-Nets for Blind Image Quality Assessment, Journal of Perceptual Imaging , vol. 4, no. 1, pp. 10402-1--10402-13, 2021.
Datei: | |
Bibtex: | ![]() @article{GrBa21a, title={{FP}-{N}ets for {B}lind {I}mage {Q}uality {A}ssessment}, author={Gr{\"u}ning, Philipp and Barth, Erhardt}, journal={Journal of Perceptual Imaging}, volume={4}, number={1}, pages={10402-1--10402-13}, year={2021}, url={https://www.inb.uni-luebeck.de/fileadmin/files/publications/inb-publications/pdfs/GrBa21a.pdf} } |
Towards Explainable Ear Recognition Systems Using Deep Residual Networks, IEEE Access , pp. 1-1, 2021.
DOI: | 10.1109/ACCESS.2021.3109441 |
Datei: | ACCESS.2021.3109441 |
Bibtex: | ![]() @article{AlLiBaIdMa21, author = {Hammam Alshazly and Christoph Linse and Erhardt Barth and Sahar Ahmed Idris and Thomas Martinetz}, journal = {IEEE Access}, title = {{T}owards {E}xplainable {E}ar {R}ecognition {S}ystems {U}sing {D}eep {R}esidual {N}etworks}, year = {2021}, pages = {1-1}, doi = {10.1109/ACCESS.2021.3109441}, url = {https://doi.org/10.1109/ACCESS.2021.3109441} } |
2020
Log-Nets: Logarithmic Feature-Product Layers Yield More Compact Networks, in Artificial Neural Networks and Machine Learning -- ICANN 2020 , Farkaš, Igor and Masulli, Paolo and Wermter, Stefan, Eds. Cham: Springer International Publishing, 2020. pp. 79--91.
Feature Products Yield Efficient Networks, arXiv preprint arXiv: 2008.07930 , 2020.
Datei: | |
Bibtex: | ![]() @article{GrMaBa20, title={Feature Products Yield Efficient Networks}, author={Gr{\"u}ning, Philipp and Martinetz, Thomas and Barth, Erhardt}, journal={arXiv preprint arXiv:2008.07930}, year={2020}, url = {https://www.inb.uni-luebeck.de/fileadmin/files/publications/inb-publications/pdfs/GrMaBa20.pdf} } |
Deep Convolutional Neural Networks for Unconstrained Ear Recognition, IEEE Access , vol. 8, pp. 170295--170310, 2020. IEEE.
Datei: | |
Bibtex: | ![]() @article{AlLiBaMa20, title={Deep {C}onvolutional {N}eural {N}etworks for {U}nconstrained {E}ar {R}ecognition}, author={Hammam Alshazly and Christoph Linse and Erhardt Barth and Thomas Martinetz}, journal={IEEE Access}, publisher={IEEE}, volume={8}, pages={170295--170310}, year={2020}, url = {https://www.inb.uni-luebeck.de/fileadmin/files/publications/inb-publications/pdfs/AlLiBaMa20.pdf} } |
A task-dependent active learning method for axon segmentation with CNNs, Proceedings on Automation in Medical Engineering , vol. 1, no. 1, pp. 025--025, 2020.
Datei: | |
Bibtex: | ![]() @article{GrPaZiBaMa20, title={A task-dependent active learning method for axon segmentation with CNNs}, author={Gr{\"u}ning, Philipp and Palumbo, Alex and Zille, Marietta and Barth, Erhardt and Mamlouk, Amir Madany}, journal={Proceedings on Automation in Medical Engineering}, volume={1}, number={1}, pages={025--025}, year={2020}, url = {https://www.inb.uni-luebeck.de/fileadmin/files/publications/inb-publications/pdfs/GrPaZiBaMa20.pdf} } |
2019
A multi-organ nucleus segmentation challenge, IEEE transactions on medical imaging , vol. 39, no. 5, pp. 1380--1391, 2019. IEEE.
Ensembles of Deep Learning Models and Transfer Learning for Ear Recognition, sensors , vol. 19, no. 19, pp. 4139, 2019. Multidisciplinary Digital Publishing Institute.
Datei: | |
Bibtex: | ![]() @article{AlLiBaMa19, author={Hammam Alshazly and Christoph Linse and Erhardt Barth and Thomas Martinetz}, title = {Ensembles of {D}eep {L}earning {M}odels and {T}ransfer {L}earning for {E}ar {R}ecognition}, journal = {sensors}, volume={19}, number={19}, pages={4139}, year={2019}, publisher={Multidisciplinary Digital Publishing Institute}, url = {https://www.mdpi.com/1424-8220/19/19/4139}, url = {https://www.inb.uni-luebeck.de/fileadmin/files/publications/inb-publications/pdfs/AlLiBaMa19.pdf} } |
Handcrafted versus CNN Features for Ear Recognition, Symmetry , vol. 11, no. 12, pp. 1493, 2019.
Datei: | |
Bibtex: | ![]() @article{AlLiBaMa19a, title={{Handcrafted versus CNN Features for Ear Recognition}}, author={Hammam Alshazly and Christoph Linse and Erhardt Barth and Thomas Martinetz}, journal={Symmetry}, volume={11}, number={12}, pages={1493}, year={2019}, url = {https://www.inb.uni-luebeck.de/fileadmin/files/publications/inb-publications/pdfs/AlLiBaMa19a.pdf} } |
2017
A Hybrid Convolutional Variational Autoencoder for Text Generation, in EMNLP , Association for Computational Linguistics, 2017. pp. 627--637.
A Review of Neural Network based Semantic Segmentation for Scene Understanding in Context of the self driving Car, 2017.
Recursive autoconvolution for unsupervised learning of convolutional neural networks, 2017. pp. 2486--2493.
Sensing Forest for Pattern Recognition, in Advanced Concepts for Intelligent Vision Systems: 18th International Conference, ACIVS 2017 , Jacques Blanc-Talon and Rudi Penne and Wilfried Philips and Dan Popescu and Paul Scheunders, Eds. Springer, 2017. pp. 126--137.
Datei: | 978-3-319-70353-4_11 |
Bibtex: | ![]() @inproceedings{BuMaBa17, author= {Irina Burciu and Thomas Martinetz and Erhardt Barth}, editor= {Jacques Blanc-Talon and Rudi Penne and Wilfried Philips and Dan Popescu and Paul Scheunders}, title = {Sensing {F}orest for {P}attern {R}ecognition}, booktitle = {Advanced Concepts for Intelligent Vision Systems: 18th International Conference, {ACIVS} 2017}, series = {Lecture Notes in Computer Science}, volume = {10617}, year = {2017}, publisher = {Springer}, pages = {126--137}, url = {https://link.springer.com/chapter/10.1007/978-3-319-70353-4_11} } |
Using CNN features to better understand what makes visual artworks special, Frontiers in Psychologie 8 , pp. 830, 2017.
2016
Learning Efficient Data Representations with Orthogonal Sparse Coding, IEEE Transactions on Computational Imaging , vol. 2, no. 3, pp. 177--189, 09 2016.
DOI: | 10.1109/TCI.2016.2557065 |
Datei: | |
Bibtex: | ![]() @article{ScBaMa16, author = {Sch{\"u}tze, Henry and Barth, Erhardt and Martinetz, Thomas}, title = {{L}earning {E}fficient {D}ata {R}epresentations with {O}rthogonal {S}parse {C}oding}, journal = {IEEE Transactions on Computational Imaging}, volume = {2}, number = {3}, month = {09}, pages = {177--189}, ISSN = {2333-9403}, year = {2016}, doi = {10.1109/TCI.2016.2557065}, url = {https://www.inb.uni-luebeck.de/fileadmin/files/publications/inb-publications/pdfs/ScBaMa16.pdf} } |
Recurrent Dropout without Memory Loss, in COLING , ACL, 2016. pp. 1757--1766.
Image classification with recurrent attention models, in SSCI , IEEE, 2016. pp. 1--7.